Coverage of FDA’s AI/ML Medical Devices Workshop - Part 3: A Summary of the Panel Discussions
In the weeks leading up to FDA’s October 14, 2021 Transparency of AI/ML Enabled Medical Devices Workshop (Workshop), we took a brief look at the history of FDA’s regulation of medical device software and the agency’s more recent efforts in regulating digital health. In this post, we will provide an overview of the topics discussed at the Workshop and our impressions of the agency’s likely next steps.
- Transparency: What Is Needed and Why?
- According to FDA, transparency is important because it helps patients and physicians make informed decisions, supports proper use of devices, promotes health equity, facilitates evaluation and monitoring of device performance, fosters trust, and promotes device adoption.
- Patients expressed the need for enough information to have informed discussions with their doctors. They want to understand how the technology works and how to use it safely, how collected information will be used and disclosed, what factors may affect accuracy, as well as any other limitations of the technology. Finally, they expressed the need to test the technology on a diverse population and in a variety of contexts in order to avoid errors in certain populations and settings, and they added that FDA should use its authority to require diverse testing data as part of the approval process.
- Manufacturers acknowledged the need for testing AI-ML enabled devices in diverse populations and settings to avoid errors that can be introduced by less diverse data sets. They also acknowledged that working with patients during development to better understand the user experience and labeling effectiveness could improve the efficacy and safety of the product. They also expressed the need for clear instructions from the agency on what should be included in device labeling and for FDA to maintain a risk-based framework for regulating such devices. Some expressed the importance of working with the existing regulatory framework for “locked” artificial intelligence and basing any augmentation of the existing framework for unlocked machine learning algorithms on the level of risk presented by the software’s intended use (e.g. what type of care is involved, and is it a tool used and interpreted by health care providers, or does the software make decisions its own).
- Health plans expressed a need for understanding of a product’s accuracy across different patient populations and care settings, how use of the technology compares to more traditional treatment or diagnostic methods (e.g. relative risk of using the technology), and the cost.
- Health care providers discussed the need for improved clinical validation and testing on diverse patient populations and charged FDA with creating a specific set of testing parameters and requirements for AI/ML-enabled devices. They also mentioned the need for sufficient detail regarding the efficacy of the technology in different patient populations (e.g. demographics and co-morbidities) and a clear indicator of whether the technology is locked or involves “continuous learning,” so they can make an informed decision on whether the technology is appropriate for their patients. Additionally, they mentioned the need for enough information to enable an informed discussion with their patients about:
- How the technology works
- How decisions are being made using the technology
- The appropriate scope of use
- Privacy and security safeguards for patient information
- What the results mean and their degree of accuracy
- How much risk is involved
- What to watch out for that indicates something isn’t working correctly
- Labeling
Stakeholders participating in the Workshop discussed what form(s) product labeling might take, and whether something analogous to a nutrition label would be helpful – in other words, a short-form, uniform label formatted to present essential, easily understandable information about the AI/ML-enabled device’s accuracy, fairness, generalization, transparency, and robustness, among other things). Patients discussed the need to have a brief overview with critical information, which might look more like a nutrition label, but they also mentioned that more information should be made readily available to patients and their providers, similar to a more lengthy package insert for prescription drugs. Stakeholders also discussed the idea that information about AI/ML-enabled devices provided to a health care provider and a patient should be different and appropriately tailored and that the opportunity to access real-time data about the technology’s accuracy and performance could significantly enhance transparency.
- Data Quality, Bias, and Equity
Topics of significant discussion amongst the stakeholders during the day-long event were concerns about data quality, bias, and health equity. Bias is a concern because AI/ML is so heavily data-driven and can result in inherent biases depending on the data set that is used to develop the technology. As a result, the stakeholders discussed the importance of data sets being representative of the intended patient population, and they agreed that health equity is a key goal to build into those data collection efforts as well. The group discussed the need for evaluations of sex-specific data, race and ethnicity data, and age, disabilities, and comorbidities in clinical testing and human factors analyses in order to improve consistency and transparency regarding safety, efficacy and usability for various groups.
- Proprietary Algorithms vs. Open Source/Coding
Another topic of interest to Workshop participants was how to provide transparency when companies are using proprietary software, and whether insisting on open source coding so patients and health care providers can see the algorithms used would make a difference. In general, there seemed to be more interest in understanding how the algorithm was trained, how it works, and how accurate it is within specific patient populations, rather than having access to the actual algorithm. However, it seems clear that open source coding is something that FDA is considering with respect to transparency.
- Design and Change Management
Stakeholders also discussed the need for design controls and the need for understanding users and incorporating human factors during the design and validation process for AI/ML-enabled medical devices. They debated the need for an intuitive user interface, which can benefit from early user involvement in the design process, as well as the potential for using predetermined change control plans. Such a predetermined change control plan could include the rationale for the update, a description of change in product claims, and a description of any changes to the software and its instructions for use. Finally, the stakeholders acknowledged that machine learning will be more challenging and likely require more of an ongoing validation and reporting process compared to artificial intelligence with locked algorithms.
- Data Protections
Patients and patient advocates continue to voice concerns about the potential for clinical and personal data to be used against patients. With the vast amount of information available about individuals from various sources, there are legitimate concerns about the use and disclosure of de-identified data sets due to the potential for re-identification. There are also legitimate concerns about the potential harm to patients from false positives and incidental findings, for example, misdiagnoses and denial of insurance coverage. Some also question whether patients are given sufficient information about what laws protect their personal and health information and what precautions will be used to protect their privacy before they agree to use the technology. Finally, there seems to be some consensus that some level of patient consent should be required before an AI/ML-enabled device may collect a patient’s data, and there is some question as to whether clicking “I accept” on a data privacy policy should be sufficient.
In closing, the recent Workshop highlighted an overarching theme that transparency means communicating appropriate information throughout the product lifecycle at the right times and taking into account different contextual factors, and FDA is seeking comments to elucidate how to accomplish such communication effectively. In particular, FDA seems to be interested in gathering perspectives from stakeholders on how to provide patient-centered transparency with the goal of communicating the risks and benefits of the technology, how to effectively and safely use it, and any information necessary for monitoring the device’s performance. Further, FDA is interested in methods to address health equity issues and to communicate the right information at the right time. Specifically, views on information to include on product labels and whether use of expanded media such as videos would be helpful to the agency. Additionally, FDA is seeking input on how to incorporate user participation in design, and how to best tailor its regulatory approach to AI/ML.
We encourage all interested parties, as FDA officials did at the close of the Workshop, to submit comments on the topic of AI/ML-enabled medical device transparency to the docket (FDA-2019-N-1185) by November 15, 2021.
Authors
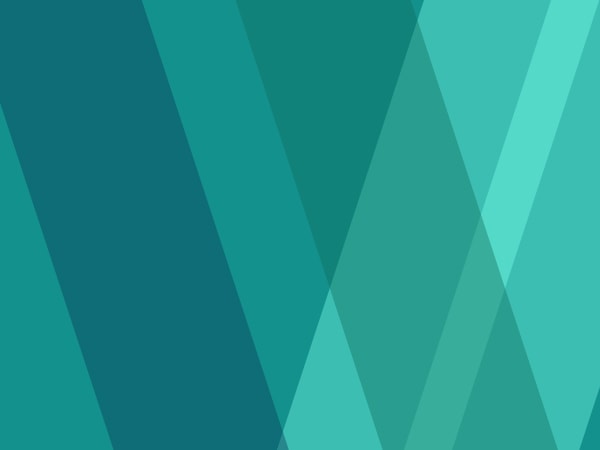