Benefits of and Best Practices for Protecting Artificial Intelligence and Machine Learning Inventions as Trade Secrets
We previously discussed which portions of an artificial intelligence/machine-learning (“AI/ML”) platform can be patented. Under what circumstances, however, is it best to keep at least a portion of the platform a trade secret? And what are some best practices for protecting trade secrets? In this post, we explore important considerations and essential business practices to keep in mind when working to protect the value of trade secrets specific to AI/ML platforms, as well as the pros and cons of trade secret versus patent protection.
Protecting AI/ML Platforms via Trade Secrets
What qualifies as a “trade secret” can be extraordinarily broad, depending on the relevant jurisdiction, as, generally speaking, a trade secret is information that is kept confidential and derives value from being kept confidential. This can potentially include anything from customer lists to algorithms. In order to remain a trade secret, however, the owner of the information must follow specific business practices to ensure the information remains secret. If businesses do not follow the proscribed practices, then the ability to protect the trade secret is waived and its associated value is irretrievably lost. The business practices required are not onerous or complex, and we will discuss these below, but many businesses are unaware of what is required for their specific type of IP and only discover their error when attempting to monetize their inventions or sell their business. To avoid this devastating outcome, we work to arm our clients with the requisite practices and procedures tailored to their specific inventions and relevant markets.
In the context of AI/ML platforms, trade secrets can include the structure of the AI/ML model, formulas used in the model, proprietary training data, a particular method of using the AI/ML model, any output calculated by the AI/ML model that is subsequently converted into an end product for a customer, and similar aspects of the platform. There are myriad ways in which the value of the trade secret may be compromised.
For example, if an AI/ML model is sold as a platform and the platform provides the raw output of the model and a set of training data to the customer, then the raw output and the set of training data would no longer qualify for trade secret protection. Businesses can easily avoid this pitfall by having legally binding agreements in place between the parties to protect the confidentiality and ownership interests involved. Another area in which we frequently see companies waive trade secret protection is where the confidential information that can be independently discovered (such as through reverse-engineering a product). Again, there are practices that businesses can follow to avoid waiving trade secret protection due to reverse-engineering. Owners, therefore, must also be careful in ensuring that the information they seek to protect cannot be discovered through use or examination of the product itself and where that cannot be avoided, ensure that such access is governed by agreements that prohibit such activities, thereby maintaining the right to assert trade secret misappropriation and recover the value of the invention.
To determine if an invention may be protected as a trade secret, courts will typically examine whether the business has followed “best practices” or “reasonable efforts” for the type of IP and relevant industries. See e.g. Intertek Testing Services, N.A., Inc. v. Frank Pennisi et al., 443 F. Supp. 3d 303, 323 n.19 (E.D.N.Y. Mar. 9, 2020). What constitutes best practices for a particular type of IP can vary greatly. For example, a court may examine whether those trade secrets were adequately protected. The court may also look to whether the owner created adequate data policies to prevent employees from mishandling trade secrets. See Yellowfin Yachts, Inc. v. Barker Boatworks, LLC, 898 F.3d 1279 (11th Cir. Aug. 7, 2018)(where the court held that requiring password protection to access trade secrets was insufficient without adequate measures to protect information stored on employee devices). If the court decides that the business has not employed best practices, the owner can lose trade secret protection entirely.
Most often, a failure to ensure all parties who may be exposed to trade secrets are bound by a legally-sufficient confidentiality or non-disclosure agreement forces the owner to forfeit their right to trade secret protection for that exposed information. Owners should have experienced legal counsel draft these agreements to ensure that the agreements are sufficient to protect the trade secret and withstand judicial scrutiny; many plaintiffs have learned the hard way that improperly-drafted agreements can affect the trade secret protection afforded to their inventions. See, e.g., BladeRoom Group Ltd. v. Emerson Electric Co., 11 F.4th 1010, 1021 (9th Cir. Aug. 30, 2021)(holding that NDAs with expiration dates also created expiration dates for trade secret protection); Foster Cable Servs., Inc. v. Deville, 368 F. Supp. 3d 1265 (W.D. Ark. 2019)(holding that an overbroad confidentiality agreement was unenforceable); Temurian v. Piccolo, No. 18-cv-62737, 2019 WL 1763022 (S.D. Fla. Apr. 22, 2019)(holding that efforts to protect data through password protection and other means were negated by not requiring employees to sign a confidentiality agreement).
There are many precautions owners can take to protect their trade secrets, which we discuss below:
Confidentiality and Non-Disclosure Agreements: One of the most common methods of protecting trade secrets is to execute robust confidentiality agreements and non-disclosure agreements with everyone who may be exposed to trade secrets, to ensure they have a legal obligation to keep those secrets confidential. Experienced legal counsel who can ensure the agreements are enforceable and fully protect the owner and their trade secrets are essential as there are significant pitfalls in these types of agreements and many jurisdictions have contradicting requirements.
Marketing and Product Development: The AI/ML platform itself should also be constructed and marketed in such a way as to prevent customers from easily discovering the trade secrets, whether through viewing marketing materials, through ordinary use of the platform, or through reverse-engineering of the platform. For example, if an AI/ML platform uses a neural network to classify medical images, and the number of layers used and the weights used by the neural network to calculate output are commercially valuable, the owner should be careful to exclude any details about the layers of the AI/ML model in marketing materials. Further, the owner may want to consider developing the platform in such a way that the neural network is housed internally (protected by various security measures) and therefore not directly accessible by a customer seeking to reverse-engineer the product.
Employee Training: Additionally, owners should also ensure that employees or contractors who may be exposed to trade secrets are trained in how to handle those trade secrets, including how to securely work on or discuss trade secrets, how to handle data on their personal devices (or whether trade secret information may be used on personal devices at all), and other such policies.
Data Security: Owners should implement security precautions (including limiting who can access trade secrets, requiring passwords and other security procedures to access trade secrets, restricting where data can be downloaded and stored, implementing mechanisms to protect against hacking attempts, and similar precautions) to reduce the risk of unintended disclosure of trade secrets. Legal counsel can help assess existing measures to determine whether they are sufficient to protect confidential information under various trade secret laws.
Pros and Cons of Trade Secret Protection over Patent Protection
Trade secret protection and patent protection are obtained and maintained in different ways. There are many reasons why trade secret protection may be preferable to patent protection for various aspects of an AI/ML platform, or vice-versa. Below we discuss some criteria to consider before deciding how to protect ones platform.
Protection Eligibility: As noted in our previous blog post, patent protection may be sought for many components of an AI/ML platform. There are, however, some aspects of an AI/ML platform that may not be patent-eligible. For example, while the architecture of a ML model may be patentable, specific mathematical components of the model, such as the weight values, mathematical formulas used to calculate weight values in an AI/ML algorithm, or curated training data, may not, on their own, be eligible for patent protection. If the novelty of a particular AI/ML platform is not in how an AI/ML model is structured or utilized, but rather in non-patentable features of the model, trade secret protection can be used to protect this information.
Cost: There are filing fees, prosecution costs, issue fees, and maintenance fees required to obtain and keep patent protection on AI/ML models. Even for an entity that qualifies as a micro-entity under the USPTO’s fee schedule, the lifetime cost of a patent could be several thousand dollars in fees, and several thousand dollars in attorney’s fees to draft and prosecute the patent. Conversely, the costs of trade secret protection are the costs to implement any of the above methods of keeping critical portions of the AI/ML model secret from others. In many instances, it may be less expensive to rely on trade secret protection, than it may be to obtain patent protection.
Development Timeline: AI/ML models, or software that implements them, may undergo several iterations through the course of developing a product. As it may be difficult to determine which, if any, iterations are worth long-term protection until development is complete, it may be ideal to protect each iteration until the value of each has been determined. However, obtaining patent protection on each iteration may, in some circumstances, be infeasible. For example, once a patent application has been filed, the specification and drawings cannot be amended to cover new, unanticipated iterations of the AI/ML model; a new application that includes the new material would need to be filed, incurring further costs. Additionally, not all iterations will necessarily include changes that can be patented, or it may be unknown until after development how valuable a particular modification is to technology in the industry, making it difficult to obtain patent protection for all iterations of a model or software using the model. In these circumstances, it may be best to use a blend of trade secret and patent protection. For example, iterations of a model or software can be protected via trade secret; the final product, and any critical iterations in between, can subsequently be protected by one or more patents. This allows for a platform to be protected without added costs per iteration, and regardless of the nature of the changes made in each iteration.
Duration of Protection: Patent owners can enjoy protection of their claimed invention for approximately twenty years from the date of filing a patent application. Trade secret protection, on the other hand, lasts as long as an entity keeps the protected features a secret from others. For many entities, the twenty-year lifetime of a patent is sufficient to protect an AI/ML platform, especially if the patent owner anticipates substantially modifying the platform (e.g., to adapt to future needs or technological advances) by the end of the patent term. To the extent any components of the AI/ML platform are unlikely to change within twenty years (for example, if methods used to curate training data are unlikely to change even with future technological advances), it may be more prudent to protect these features as trade secrets.
Risk of Reverse-Engineering: As noted above, trade secrets do not protect inventions that competitors have been able to discover by reverse-engineering an AI/ML product. While an entity may be able to prevent reverse-engineering of some aspects of the invention through agreements between parties with permission to access the AI/ML product or through creative packaging of the product, there are some aspects of the invention (such as the training data that needs to be provided to the platform, end product of the platform, and other features) that may need to remain transparent to a customer, depending on the intended use of the platform. Such features, when patent-eligible, may benefit more from patent protection than from trade secret protection, as a patent will protect the claimed invention even if the invention can be reverse-engineered.
Exclusivity: A patent gives the patent owners the exclusive right to practice or sell their claimed inventions, in exchange for disclosing how their inventions operate. Trade secrets provide no such benefit; to the extent competitors are able to independently construct an AI/ML platform, they are allowed to do so even if an entity has already sold a similar platform protected by trade secret. Thus, to the extent an exclusive right to the AI/ML model or platform is necessary for the commercial viability of the platform or its use, patent protection may be more desirable than trade secret protection.
Conclusion
Trade secret law allows broad protection of information that can be kept secret from others, provided certain criteria are met to ensure the information is adequately protected from disclosure to others. Many aspects of an AI/ML platform can be protected under either trade secret law or patent law, and many aspects of an AI/ML platform may only be protected under trade secret law. It is therefore vital to consider trade secret protection alongside patent protection, to ensure that each component of the platform is being efficiently and effectively protected.
To learn more from the Mintz IP team, follow us on LinkedIn and check out our Exclusive Rights podcast.
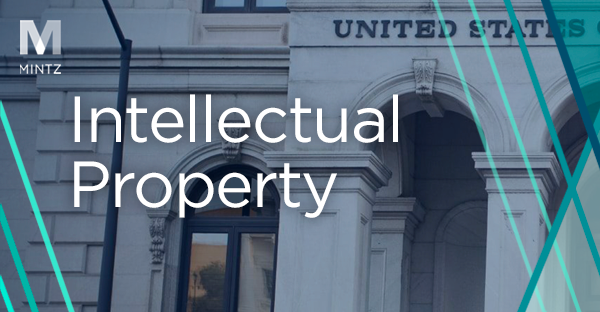