AI in Biotech and Synthetic Biology: What Can Be Protected? What Should Be Kept Secret?
Machine learning (ML), bioinformatics, artificial intelligence (AI), and other computational tools have become ubiquitous in the biotech and synthetic biology industries because such technology allows for rapid processing of a large amount of complex data to produce advancements in therapeutics and diagnostics. As we previously discussed in Patenting Considerations for Artificial Intelligence in Biotech and Synthetic Biology Part 1 and Part 2, applications such as sequencing and functional genomics; drug design, discovery, and testing; pharmacology; big data analytics; cancer diagnosis; and target identification and designing constructs, have realized tremendous benefits due to the use of machine learning and AI. Thus, as the landscape becomes increasingly more competitive, it is important for companies, particularly in the aforementioned industries, to obtain patent protection for their AI-related technology.
Applying for patent protection presents certain risks, especially for computer-based inventions. As previously discussed here and here, there are a number of ways to mitigate those risks. Importantly, when drafting patent applications, it can be beneficial to describe the advantages of the inventive technology to show how the invention is used in a practical application to solve a technical problem, and to disclose as many technical details as possible about the inventive technology.
In the case of AI-based inventions, companies generally seek to protect the use or particular application of their ML model. While the use of the ML model is a key aspect for a company to protect, it is far from the only one. In an effort to disclose as many technical details as possible, companies should consider protecting all facets of their AI-related technology, including the particular practical application of their ML model; the training of data supporting their ML model; the architecture of their ML model; the end product or result of processing data using their ML model; the preparation for clinical trials using the end product or result; and the user interface for presenting the result, among others. With that in mind, companies should still carefully consider whether any of these aspects should be kept as a trade secret, especially if those aspects are not readily discernible.
1. Use of the ML Model
One of the most important parts of AI-related technology for companies to protect is the use of the companies’ ML model. This includes new approaches to using the ML model and processing data in a practical application to solve particular problems. For example, companies may protect their innovative methods of processing data using ML models to diagnose or predict diseases or other abnormalities in patients, analyze the health of the patient, assess side effects of a medication, discover drugs, etc.
Even when a particular solution relies on off-the-shelf or open-source ML models, the novel use of the ML model may still be afforded patent protection. In those instances, however, it is especially important to describe, in the patent application, how the ML model is specifically tailored to the particular application. As an example, an off-the-shelf ML model may be used to predict, based on a set of images, whether a patient is likely to have cancerous tissue. While an off-the-shelf ML model is used in this example, a strategically drafted patent application may allow companies to still obtain patent protection by providing specific details about how its approach is different from and/or better than generic image processing techniques. Patent protection can also be obtained if the off-the-shelf ML model is optimized in a specific manner, such as by stacking multiple off-the-shelf ML models in a way that is particularly efficient to achieve a desired result.
2. Training Data for the ML Model
Innovation in the field of AI is not merely about the end result. The datasets used to train the ML models are just as important, as ML models perform better when they are effectively trained using more accurate data. As an example, certain ML models can identify target disease biomarkers. The training data for those ML models may include features of proteins that have been labeled, classified, or gathered in a unique way. The training data may also include synthetic data previously generated by that same ML model or another ML model.
Companies can thus obtain patent protection for various aspects of the training data, such as:
-
how the data is prepared, enriched, anonymized, or represented;
-
how the ML model is trained using the training data;
-
how synthetic training data is generated to supplement the volume and/or variety of available training data; and
-
how the training data is tested, evaluated, or validated.
As to the training data itself, companies may consider trade secret protection, rather than patent protection, for the specific subset of data used to train the ML model if the data is not apparent from the ML model or use of the ML model. The data would need to be kept secret, and companies would need to implement various affirmative protocols for maintaining the data as a trade secret.
3. Architecture of the ML Model
Rather than use an off-the-shelf ML model, companies may develop a custom ML model to achieve a desired result. During development of the ML model, companies may add new components or layers to the architecture of the ML model. In the context of the type of ML model, such as a supervised learning model, unsupervised learning model, reinforcement learning model, neural network, and the like, the new components or layers, or the location of the new components or layers may be patentable.
During development of an ML model, weights, coefficients, biases, or other parameters may be assigned to the inputs of the ML model. While patents can protect novel arrangements and the new components or layers of the ML model, the weights, coefficients, biases, or other parameters may be more suitable for trade secret protection.
4. End Product of the ML Model
As discussed above, ML models may be used to achieve a desired result or end product. That end product may include a prediction, a classification, a discovery, or another insight. The end product may also be deployed as part of medical equipment or a medical device. While the ML model (or AI) itself cannot be an inventor, new products, such as new drugs, therapies, and representations identified or developed based on the results of the ML model, as well as the apparatuses deploying the end product, may still be patented.
5. Preparing for Clinical Trials using the End Product
Generally, drugs or other end products, whether or not the drugs or other end products were developed based on an ML model, may be tested and/or validated during clinical trials. Selecting the proper subjects for the clinical trials may be difficult, as clinical trials may require a particular number of subjects within a desired subset of a population. ML models may be used to select subjects for the clinical trials. When ML models are used to select subjects for clinical trials, companies should consider protecting the various aspects of those ML models.
6. Presentation of data
Often, the results of the ML model may be presented in a unique manner via a user interface. The results of one or more ML models may be combined and then also presented via a dashboard of the user interface. Multiple types of patent protection are available for the presentation of such data. Companies may protect the functional aspects of presenting the generated data by filing a utility patent application. Should the data be presented in a visually distinct manner, companies may also consider protecting the ornamental aspects of the presentation by filing a design patent application. Strategically crafted patent portfolios may include both utility and design patent applications.
Accordingly, patent protection should be considered for all aspects of a company’s ML model and AI-related technology.
To learn more from the Mintz IP team, follow us on LinkedIn and check out our Exclusive Rights podcast.
Authors
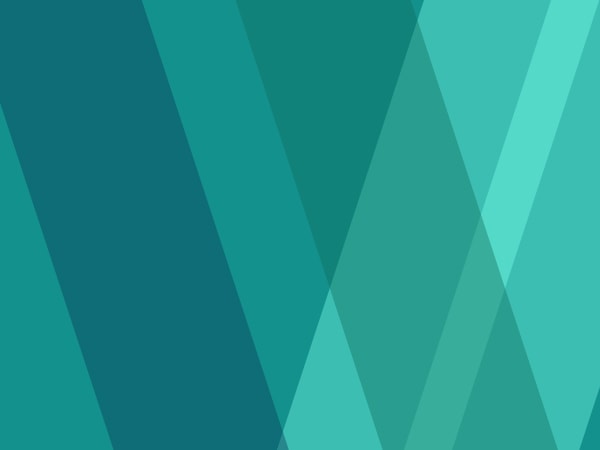